Visual Development and Object Recognition
116
Learning Objectives
Know what Bayesian inference is.
Know why Bayesian inference is important in visual perception.
Bayesian inference comes from Thomas Bayes (1702-1761) who described how inference occurs. Inference based on the probability of an event occuring, using the probability of an event or observation is described using p(A|B) x p(B) = p(B|A) x p(A). We calculate the probability of something developed from prior experience p(S). Bayesian inference can be understood by looking at the Boston Dynamic Big Dog (Fig.11.9.1). Since it appears to be a dog, we use prior experience multiplied by the generative model (living things move) to infer that it is alive.
Visual perception is affected by a combination of things. The human brain uses the image it’s being given to calculate the posterior likelihood that it is seeing a specific scene. This is done by using the formula; p(S|I) = p(I|S) x p(S)/p(I) where S is the scene and I is the image. p(I|S) is called the generative model. This is the probability that the scene will generate a given image. p(S) is the probability of a particular scene which comes from prior experience. p(I) is the probability of the image. This image will most likely not change based upon how the brain interprets the scene, so when calculating the posterior likelihood of perceiving a specific scene, it can be dropped from the calculation. This leads to p(I|S) being proportional to p(I|S)*p(S). That is to say the probability that a scene will generate a particular image increases or decreases depending on how familiar the person viewing it is. An example of this can be seen when viewing the Boston Dynamic Big Dog (Fig.11.9.1). If, for example, somebody were to kick the dog, the viewer would have a negative emotional reaction. This is because the viewer perceives the dog as alive. This is because even though they’ve never seen an animal that looks like the dynamic big dog, it still moves like a living thing. The generative model (p[I|S]) asks the probability of the movement being generated by a living thing. Even though the animal looks nothing like a real dog, the movement overrides it and the human brain will perceive it as a living organism.
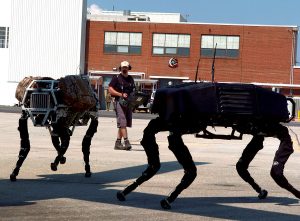
Cheryl Olman PSY 3031 Detailed Outline
Provided by: University of Minnesota
Download for free at http://vision.psych.umn.edu/users/caolman/courses/PSY3031/
License of original source: CC Attribution 4.0
Adapted by: Elton Wang and Cole Lachmiller